Modern data management can enable organizations to make better decisions and gain the necessary capabilities to address current operational challenges. In this blog, we'll discuss what modern data management entails and what are some of its best practices.
What is Modern Data Management?
In today’s knowledge economy, few organizations would debate that data is now one of their most important assets. It is now often critical to service delivery at many organizations. Processing it too late can be disastrous. Similarly, seeing it stolen or compromised can be immensely destructive. Just like any asset, it needs to be managed with care.
Data management is about managing the way that is collected, stored, protected, and processed to optimize operations and assist decision-making. As the requirements and organizational needs change, and the volume of data generated and processed by organizations continues to grow, the approaches to data management have undoubtedly had to modernize to adapt.
Modern data management refers to all the practices that help streamline and improve traditional data management processes in order to address the challenges of today and prepare organizations for the future ones, and help organizations fully leverage the value of their information.
Characteristics of Modern Data Management
Modern data management has the following features and characteristics:
- User-centric. Collecting data for data’s sake adds little value. The data strategy should reflect organizational objectives and be led not by the IT department but by business users themselves. Systems should reflect the needs of the users they are serving. Different teams and people fill different roles. As such, your enterprise data management platform and approaches should deliver different processes for different user personas.
- Secure. As more information is digitized, the risks of theft or leaks are undoubtedly greater. A modern data management approach puts security at the forefront, not only in terms of infrastructure or software-level cybersecurity provisions but also carefully considered data governance models, ensuring only the users that need certain data to deliver their functions have access to them.
- Flexible. Organizations have to remain flexible to stay relevant and competitive in today’s fast-changing world. This doesn’t just apply to their value propositions in the market but also their internal systems and procedures. If any change in business strategy implies heavy costs in time and money on changes to enterprise data management platforms, our ability to be agile will be severely limited.
Check our blog on How No-Code Development is Driving Digital Transformation
- Centralized. Under modern data management thinking, data silos should be a thing of the past and mitigated. Disconnected systems not only generate duplication but also confuse and slow down the ability of an organization to have a single version of the truth which can drastically affect our ability to respond to challenges and preempt issues.
- Cloud-first. Data should be accessible regardless of where the people that need it find themselves at the time they need to make a decision. Provided security is well-considered, modern organizations should always deliver data where it’s needed. Cloud-native solutions not only allow this, but they also facilitate our ability to scale without significant hardware blockers or delays.
- Cost-Optimized. Applying cost optimization techniques to a modern data management program is crucial to reduce costs, maximize the business value, and increase the operational efficiency of all data management initiatives.
Best practices to Achieve Modern Data Management
To achieve modern data management in your organization, we recommend the following ten best practices:
- Define goals
What do we want to achieve? It should be crystal clear what our high-level aspirations are with regard to data before we invest in a system to support those ambitions. What metrics are we trying to maximize or minimize? How do we want to measure them? What sort of analysis would help refine our approach over time? What sort of processes do we want to digitize, and why? - Start simple and build-up
Investing in a new enterprise data management platform can be daunting at first. Where do you start? We recommend starting small with use cases that are clear to you now and working up from there adding more teams, functions, processes, and metrics over time. A modern system should allow you to build over time and iterate easily without generating extra costs.
- Don’t cut corners on metadata
As you develop data architectures for you, your team, and your use cases, it’s a good idea to invest in clear naming conventions for folders and data tables, unique identifiers for data records (e.g. 1, 2, 3 vs IR1, IR2, IR3 for Incident Reports) as well as descriptions for your data tables and data fields. This will ensure clear documentation for a more sustainable system that can continue to function well despite changes in human resources over time.
- Data quality: Garbage in, garbage out
Give careful attention to how you design data collection instruments to ensure they minimize the potential for human error and stay relevant despite changes in other parts of the systems. Guiding users through data entry with clear validation rules, fixed dropdown lists, or better yet dynamic links or references to other related databases is one way to ensure data collected is of the utmost quality.
- Audit trail: Understand what happens to your data over time
Having a single version of the truth easily accessible to those who need it is great but sometimes being able to see the history of changes can be key for compliance and security considerations as well as for optimal service delivery. If knowing who did what when to the data is critical, make sure to invest in systems that keep track of changes otherwise you’ll be disappointed later on to find there’s no way to capture that data retroactively.
- Self-service analytics
Collecting and storing data in and of itself is one thing but if it’s not processed for insights it won’t do much good. Historically, analytics was reserved to tech-savvy analysts (business intelligence analysts or engineers) who would deliver on requests from non-technical, business users. This is a thing of the past. Not only is it inefficient (creating unnecessary and costly bottlenecks), the delays generated by the old model can have significant negative repercussions on your business metrics. As such we recommend investing in systems that allow for self-service analytics.
- A balance between accessibility and security
You don’t want security measures to prevent people who need data to perform their duties from accessing the data. Similarly, you don’t want data to be so accessible that everybody across the organization has equal access despite 99% of users not requiring access to it. Striking a balance here is key and that’s easier to achieve when the data governance and access permissions models are precise (micro vs macro). It should be clear whether a user or group of users need to create, read, update or delete (CRUD) access to a given dataset, and roles and permissions assigned should reflect their needs.
- Carefully review cybersecurity provisions of systems used
Cloud infrastructure is preferable for accessibility and access but they don’t all come equal. ISO27001-certified infrastructure should be the minimum but we strongly encourage you to look for solutions that encrypt data not just in transit (SHA-256 bit SSL) but also at rest. Be wary of working off of patchwork of SME-focused applications as they can increase your risk exposure and decrease your control.
- Factor in localization requirements
More and more organizations are international, operating across borders with multinational teams. English is the lingua franca of business but complex, multi-party information flows may require localized versions of systems to collect data from broader groups of stakeholders inside and outside the organization. Investing early in systems that allow for user-managed translation can be a blessing later when you look to scale the platform to users who work in Spanish or Arabic.
- Invest in platforms that drive modern data management practices
Make sure the systems you invest in are easily adapted to your needs and can grow with you as your needs evolve. If data management will be a critical part of your organization going forward, you will want to work with partners that can guarantee uptime and deliver support that removes worries from your day-to-day and gives you peace of mind.
Adopting a Modern Enterprise Data Management Platform
All data management will vary depending on your business, industry, and specific data goals, and it is critical that you find a data management platform that is best suited for your business and delivers modern data management capabilities.
Investing in a modern enterprise data management platform may require an upfront investment but it will pay itself back many times over in the long run.
Looking for the right tool?
AKTEK iO can help organizations like yours achieve their data goals with an end-to-end enterprise data management platform that delivers modern and agile data management capabilities. Request a demo and learn more about AKTEK iO.
Some of our sources:
- https://tdwi.org/articles/2021/06/21/diq-all-best-practices-to-modernize-your-data-management.aspx
- https://www.dataversity.net/seven-data-management-tips-and-best-practices-for-small-business-owners/#
- https://www.cio.com/article/3604333/7-tips-for-modernizing-data-management.html?upd=1627053297710
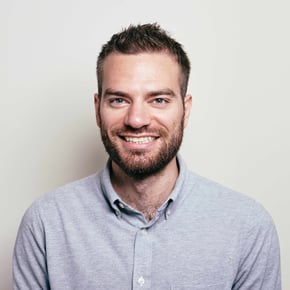